Beyond Due Diligence: How AI-Enhanced KYB is Reshaping AML Practices
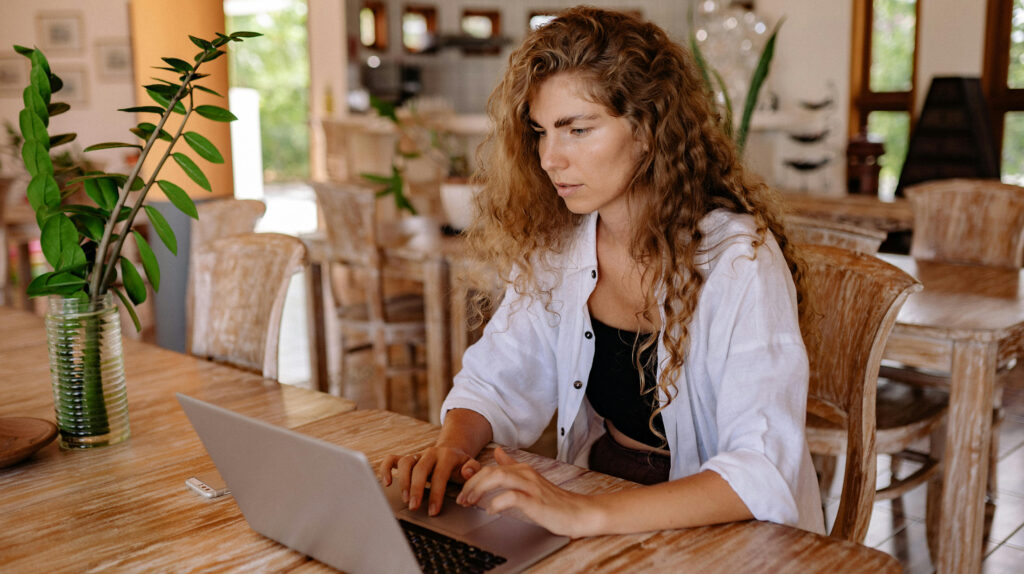
Table of Contents
The interconnected and digital nature of today’s global financial system has amplified the challenges of money laundering and terrorism financing. These constantly evolving threats demand a fundamental shift in our countermeasures, as conventional approaches, based on manual reviews and rigid rule systems, are struggling to keep pace with increasingly sophisticated criminal tactics.
This article explores how AI-enhanced KYB is improving and fundamentally reshaping the global approach to AML compliance.
The AI Revolution in Know Your Business (KYB)
AI-enhanced KYB represents a paradigm shift from conventional, often manual due diligence processes to dynamic, intelligent systems capable of processing vast amounts of data in real-time. This approach leverages advanced technologies such as machine learning, Natural Language Processing (NLP), and predictive analytics to transform raw data into actionable insights.
The global AI in fintech market size was valued at USD 9.45 billion in 2021 and is expected to grow at a Compound Annual Growth Rate (CAGR) of 16.5% from 2022 to 2030 (Grand View Research). Industry statistics underscore the adoption of AI in AML, which looks set to continue.
Transforming Traditional Due Diligence
AI-driven technologies are redefining the due diligence landscape, shifting from reactive, checklist-based approaches to proactive, dynamic risk assessment methodologies that adapt in real-time to the ever-changing financial ecosystem.
1. Enhanced Data Collection and Analysis
AI-driven KYB systems go beyond manual data gathering, employing advanced algorithms to:
- Automatically collect and process data from diverse sources in real-time
- Utilise NLP to extract relevant information from unstructured text
- Analyse millions of data points across multiple languages for a global perspective
2. Advanced Risk Assessment Models
AI introduces:
- Dynamic risk profiling that evolves based on new information
- Multi-dimensional risk assessment considering a wide range of factors
- Customisable risk models tailored to specific industry requirements or regulatory frameworks
3. Predictive Analytics in KYB
AI’s predictive capability enables:
- Pattern recognition to identify trends indicating future risks
- Behavioural analysis to anticipate changes in a business’s risk profile
- Scenario modelling to prepare for various risk eventualities
The Impact on AML Practices
Integrating AI into AML practices catalyses a paradigm shift in how financial institutions detect, prevent, and respond to illicit activities. This shift fundamentally alters compliance efforts’ speed, scope, and effectiveness across the global financial sector.
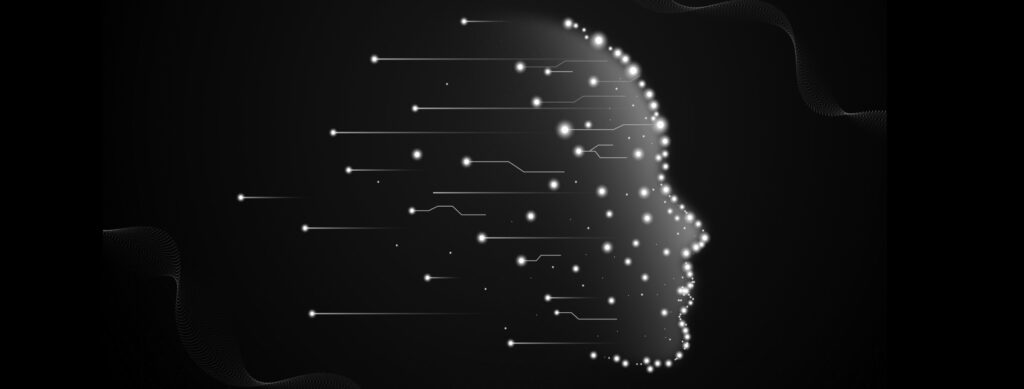
1. Machine Learning Models
Machine learning models distinguish between genuine risks with increasing accuracy over time. Compared to traditional rule-based systems, this reduces false positives by up to 30%
2. Contextual Analysis
AI systems can perform deep contextual analysis of transactions and behaviours, considering a more comprehensive range of factors than traditional rule-based systems. This holistic approach allows for more nuanced risk assessments, reducing false positives while capturing more genuine risks.
3. Anomaly Detection
Advanced AI algorithms excel at detecting subtle anomalies that conventional systems or human analysts might miss. By analysing vast amounts of data and establishing complex patterns, AI can identify truly suspicious activities with greater precision.
4. Continuous Learning and Adaptation
Unlike static rule-based systems, AI models in AML continuously learn from new data and feedback, improving accuracy. This adaptive nature allows AI systems to quickly adjust to new money laundering techniques and evolving financial crime patterns.
These advancements in accuracy and false positive reduction improve AML processes’ efficiency and enhance financial crime detection’s effectiveness overall. Financial institutions can allocate their resources more effectively in the fight against money laundering by focusing human expertise on genuinely suspicious cases.
Regulatory Perspectives on AI in AML
Regulatory bodies are increasingly recognising the potential of AI in enhancing AML efforts:
- The Financial Action Task Force (FATF) released guidance in 2021 on the use of AI in AML/CFT, emphasising its potential to improve the effectiveness of AML programs.
- The European Banking Authority (EBA) published guidelines 2022 on using machine learning for AML/CFT purposes, providing a framework for responsible AI adoption.
Ethical Considerations and Responsible AI Implementation
While AI significantly benefits AML practices, the industry recognises the importance of addressing ethical considerations. Financial institutions and technology providers are implementing robust measures to ensure responsible AI use:
1. Data Privacy and Protection
Challenge:
AI systems require vast amounts of data, raising concerns about privacy.
Solutions:
- Implementation of advanced data anonymisation techniques
- Adoption of federated learning, allowing AI models to be trained across multiple decentralised datasets without exchanging raw data
- Strict adherence to global data protection regulations like GDPR and POPIA
2. Mitigating Algorithmic Bias
Challenge:
There’s a risk of perpetuating or amplifying existing biases in historical data
Solutions:
- Regular audits of AI models for potential biases
- Diverse data sourcing to ensure representative training sets
- Implementation of fairness constraints in AI algorithms
- Collaboration with ethicists and diverse stakeholders in AI development
3. Enhancing AI Explainability
Challenge:
The “black box” nature of some AI algorithms can make it challenging to explain decisions
Solutions:
- Development of Explainable AI (XAI) techniques
- Use of model-agnostic interpretation methods like SHAP (SHapley Additive exPlanations)
- Creation of intuitive visualisation tools for AI decision paths
- Regular reporting and documentation of AI decision-making processes for regulatory compliance
4. Balancing AI and Human Expertise
Challenge:
There’s a risk of over-reliance on technology, potentially diminishing human expertise
Solutions:
- Implementation of human-in-the-loop systems, combining AI efficiency with human judgment
- Continuous training programs for compliance professionals on AI capabilities and limitations
- Development of AI systems designed to augment rather than replace human decision-making
- Regular assessment of AI performance against human expert benchmarks
5. Ensuring Regulatory Compliance
Challenge:
Keeping AI systems aligned with rapidly evolving regulatory requirements
Solutions:
- Collaboration with regulatory bodies to develop AI governance frameworks
- Implementation of agile AI systems capable of rapid updates to align with new regulations
- Regular compliance audits of AI systems
- Participation in regulatory sandboxes to test and refine AI applications in controlled environments
The Future of AI in KYB and AML Practices
As AI technology continues to evolve, we can expect even more advanced applications:
- Quantum computing may enable more complex risk modelling
- Advanced biometrics could further enhance identity verification processes
- Blockchain technology might be integrated for more secure and transparent due diligence processes
- Federated learning could allow institutions to collaborate on AI models without sharing sensitive data
As financial criminals grow increasingly sophisticated, AI-enhanced KYB is becoming not just an option but necessary for organisations committed to robust AML practices. However, its implementation must balance human expertise with ethical considerations to ensure responsible and effective use.
AI-enhanced KYB is not just an improvement on traditional due diligence; it’s a fundamental reimagining of how financial institutions approach AML compliance. By leveraging the power of AI, Financial Institutions (FIs) can achieve unprecedented accuracy, efficiency, and adaptability in their KYB processes and stand at the cusp of a new era in the fight against money laundering and related financial crime.
RelyComply offers enterprises an end-to-end AML solution. Let’s show you how
Disclaimer
This article is intended for educational purposes and reflects information correct at the time of publishing, which is subject to change and cannot guarantee accurate, timely or reliable information for use in future cases.