Payment screening: To pay or not to pay
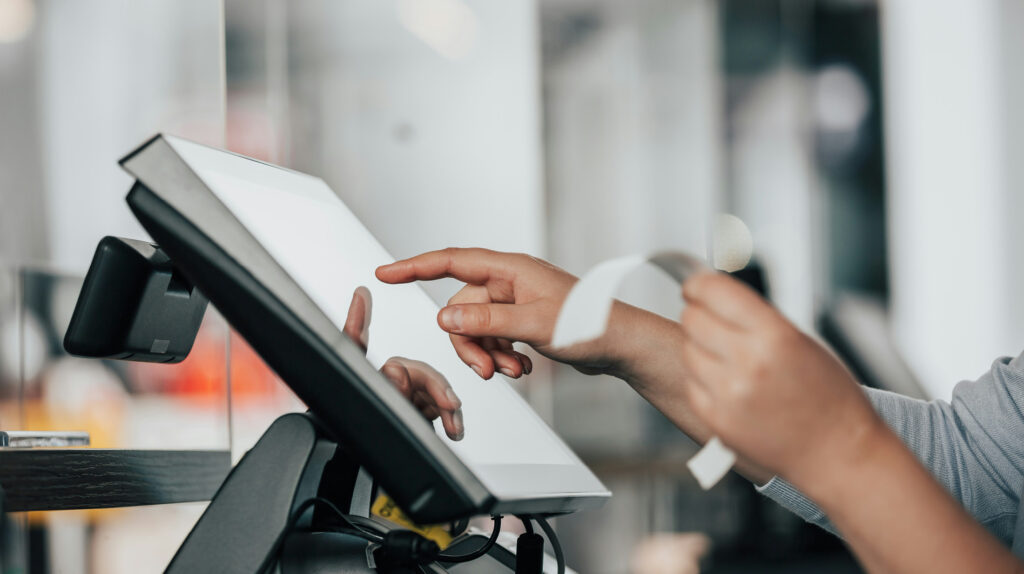
Doing business with a known criminal is bad for business. At least, if you’re a reputable company looking to keep your reputation intact. But, what if it is your customer who is engaging in financial activity with a suspicious party?
Take, for instance, the recent US sanctioning of a number of ISIS members operating economically within South Africa, thereby funding terrorist activity. Transactions which benefit such nefarious causes simply can and must be stopped. This is where the need for an intelligent payment screening process arises.
Putting practices into place to detect sanctioned or embargoed payments is not merely a matter of checking the regulator’s compliance box. It is a balancing act, taking into account the time and money costs associated with compliance and weighing this against the financial and reputational risks of doing business with the wrong crowd.
The situation is complicated by the reality of today’s payment ecosystem: staggering volumes of instant digital currency transfers across a variety of payment providers that circle the globe. Payment screening AML (anti-money laundering) solutions, which hone in on cross-border payments, need to be fast and they need to be effective.
It almost goes without saying in this day and age, but this is where smarter technology and artificial intelligence (AI) has changed the playing field. As compliance teams increasingly turn payments sanction screening over to machine learning algorithms, it seems that financial enterprises, both big and small, tend to agree.
Sanctions, of course, prohibit economic interaction with certain individuals, entities or countries that are in breach of international law or conduct. Financial institutions are under legal obligation to comply with these regulations, where payment screening identifies transactions with such parties. The process is complex, taking into account a variety of national and international sanctions lists, such as those held by the Office of Foreign Assets Control and the European Union.
PEP (politically exposed persons) screening and various financial blacklists also need to be considered to gain a holistic picture of the backdrop of financial crimes and unscrupulous business conduct. Naturally, these lists are in constant flux, calling for AML systems to be ever up-to-date. Fortunately, software automation has the capability to pull disparate data sets in real-time, constantly revising and compiling accurate sanctions lists against which to compare payments activity.
Another issue which arises when it comes to payment screening is that of speed. No one likes to wait. Customers waiting during the payment process are certainly no exception. A payment screening AML solution simply has to have low latency, which is a measure of the delay while data is processed. The goal is for high volumes of data to be processed with exceptional speed.
As an example, automatically screening a payment with our solution takes less than a second at the 99th slowest percentile. This way, payment checking is performed in near real-time with money moving quickly, regardless of the volume of transactions.
The challenge of positive matching is another minefield, one where the input of AI is perhaps most acutely felt. False positives, where a payment is flagged erroneously, cost time and money. It may be a case of an identical or misspelled name, or misinterpreted data, but whatever the reason for an alert, a compliance team member is required to investigate whether it is indeed the sanctioned party. In the event of an accurate match, this is worth the effort. Yet, false positives flood compliance teams, wasting resources and potentially angering customers should payments be delayed.
On the other hand, failing to identify positive matches implies missing out on an illegal or embargoed payment, where the consequence is potential fines and reputational damage. Machine learning is critical for both false and omitted positives, pulling in historic data from diverse sources, clarifying personal information errors and disambiguating individuals with the same names. It is a complicated technology to explain, but the long and short of it is that advanced algorithms not only radically reduce false positives, but maximise the chances that a payment to a sanctioned party will actually be flagged.
It’s about protecting your company in a world increasingly inundated with shady transactions and business relations. No one wants to be that company that helped finance illicit and sanctioned activities.
With our AI-driven payment screening suite, we can guarantee that your business will stay out of this particular limelight.
Disclaimer
This article is intended for educational purposes and reflects information correct at the time of publishing, which is subject to change and cannot guarantee accurate, timely or reliable information for use in future cases.